As part of their digitalization, organizations in all sectors are changing their processes, tools and use of their information systems. Harnessing data opens up a host of opportunities, but before thinking about the system around the data, we need to ensure its quality. According to a 2020 study by Gartner, the average cost of poor data quality could amount to €11M per year for an organization. This subject, whose importance no longer needs to be proven, also applies to alternative investment players.
The importance of data quality and data management
The alternative investment sector is currently undergoing rapid change and faces numerous challenges ranging from its operating model to the role it must play in society. To maintain this dynamic growth, the industry will have to reinvent itself. Faced with this major development, AssetValue Consulting is structured to provide a strong response. We capitalize on the expertise of experienced consultants, the know-how acquired through our projects as well as a strong positioning in the mastery of the profession of alternative investment, project management and new innovative technologies. We have strengthened our positioning through strategic partnerships with vendors of private assets solutions to offer a perfectly suited support to the needs of businesses.
Data quality: a necessity in a highly regulated environment
Data quality refers to the intrinsic characteristics of data. These include accuracy, completeness, consistency, validity, timeliness, integrity, clarity and security. Data quality can be degraded at the level of data description (static fund data, contact data, object name conflicts or imprecise object definitions) and at the level of the data itself (cashflow and performance data, null values, duplicates, abnormal values, obsolete data, etc.).
Data quality criteria
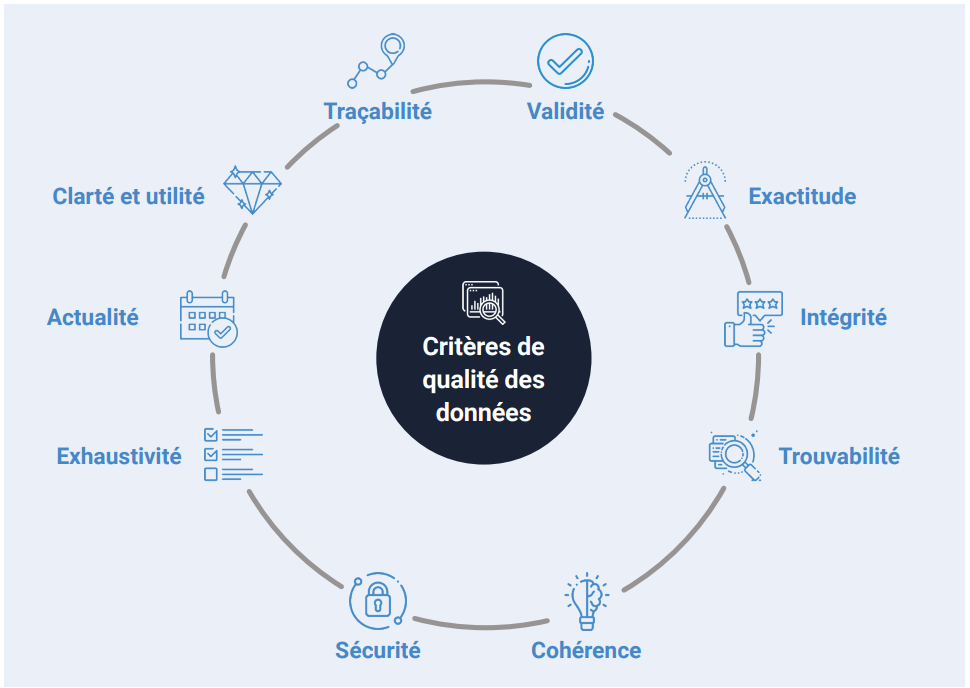
According to a survey conducted by DataValue Consulting in 2022, 37% of decision-makers consider data quality to be a major hindrance to their business. This trend is growing in a highly regulated sector such as asset management. Whether for traditional or alternative investments, numerous legal frameworks are required: from the Autorité des Marchés Financiers (AMF) to the Alternative Investment Fund Managers Directive (AIFM), via classic regulations such as AML (Anti-Money Laundering), CRS (Common Reporting Standard) or GDPR (General Data Protection Regulation). The processes involved in collecting, transforming, sharing and leveraging the data required for the alternative investment business are a strong incentive for industry players to monitor data quality. Legislation evolves rapidly, and reporting must keep pace with it. Many asset management companies have set up a data management process based on best practices and data quality measurement and control techniques. But these data management processes often remain compartmentalized, respecting existing organizational silos, and are not cross-functional within management companies. At AssetValue Consulting, we are convinced that only an organizational approach can improve data quality, by adapting each type of data (Investors, Deals, Investments...) to the quality issues at stake. This approach must be based on 4 pillars: strategy, processes, people andsupervision/continuous improvement. The data quality management process should be iterative, based on a "by design" upgrade of data quality towards a standard level of quality defined in the data strategy. This means defining upstream processes to prevent non-quality, and downstream processes to remedy anomalies.
> ALSO READ : Asset management: how to secure your data?
The road to data quality
There are four main steps involved in setting up an effective data quality management process:
- Define data, its nomenclature and its management model,
- Assess data quality in line with business expectations,
- Measure the impact of data non-quality on business processes,
- Improve data quality through data acquisition and updating processes
In the course of its experience, AssetValue Consulting has been able to confirm its convictions on the importance of implementing a data quality strategy. Any player planning to implement such a strategy must take these seven points into account to ensure the success of any large-scale project.
1. Implementing a data quality strategy requires mobilizing the entire organization, changing the way it works and its working habits. A change management component must be taken into account.
2. Functional scopes must reconcile regulatory issues, Front, Middle and Back Office business impacts and available budget.
3. The implementation of a "high value-added and economic" strategy focuses on important data perimeters (investors' personal data, data on investment positions, etc.).
4. Quality and coherence objectives must be differentiated.
5. Data quality is to be defined on a targeted perimeter via " quick wins " as part of a long-term vision.
6. Information systems must be built around an end-to-end data quality control process (CRM, Workflow, 4 eyes, MDM, automated control rules, control reporting, compliance rules, etc.).
7. To guarantee data quality over the long term, we need to build a transformation path that capitalizes on each stage. Transformation must be gradual, based on a reproducible industrial model:
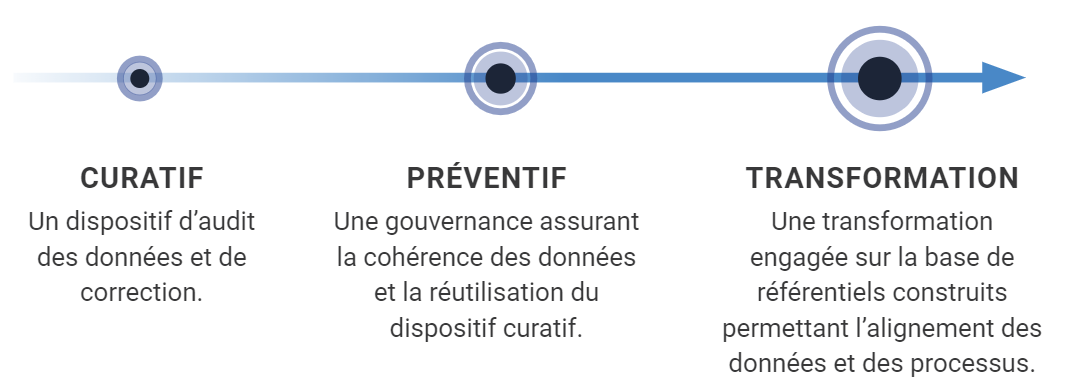
Conclusion
Data quality is a fundamental foundation for the digitalization of alternative investment players. We can't wait to make this a priority, not just for data or IT teams, but for all information system users. Maintaining data quality depends to a large extent on global awareness and sensitization. A data quality strategy will be effective and successful if all players have the capacity to guarantee this quality at their level, as the volume of data managed by the organization grows.